🔍 Predictive Analytics: Transforming Maintenance Operations
- pabloramirezgarcia
- Aug 8, 2024
- 4 min read
Predictive analytics mines existing data, identifies patterns and helps companies predict what might happen in the future based on that data. It uses predictive models that make hypotheses about future behaviours or outcomes. (IBM, 2024)
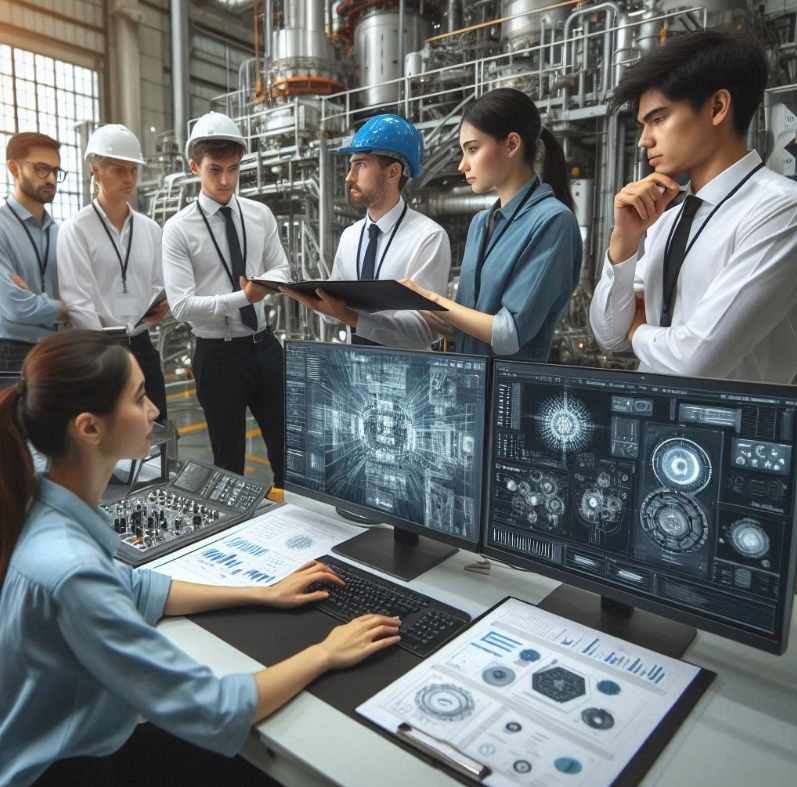
Generated with AI
What is PDM and what are the benefits of using analytics?
PdM is a proactive maintenance technique that utilizes asset data (real-time and historical) in order to determine whether the asset will fail in the future. This is performed by (1) gathering all the relevant data and predicting their future values and (2) performing anomaly detection on the predicted values to intercept potential incidents before they actually happen. Such a provision allows mitigation measures to be initiated to restore the equipment to its full operational status.
Rule-based PdM is carried out on the basis of hard-coded threshold rules, and an alert is sent if a measurement crosses the predefined thresholds, while in Machine learning (ML)-based PdM Advanced analytics are used to forecast when the next failure will occur, and, in response, early-stage maintenance is scheduled.
PDM is clearly improved by the use of data and analytics, as confirmed by several researchers, Chen et al. (2020); Schmitt et al. (2020), maintenance decision support systems (DSS), leveraging IoT, Big Data, and Machine Learning (ML), ensure equipment reliability by converting vast datasets into actionable intelligence, optimizing maintenance schedules, and predicting failures.
Advanced analytics software enhances uptime, quality, and throughput by providing insights. It suggests parameters to improve efficiency and reduce waste through real-time data analysis, and adjustment of process variables for ongoing optimization. These applications are diverse. For instance, R. Azmi et al. (2024) highlighted various benefits in research of predictive analytics in the oil and gas (O&G) industry. One notable application is optimizing drilling operations, where predictive analytics helps detect and identify drill pipe stuck-up events. Additionally, it aids in exploration and production by classifying events to minimize downtime, reduce maintenance costs, and prevent damage to installations in oil wells. In the F&B sector, unexpected equipment failures can cause product quality issues, production downtime, and increased maintenance costs. Advanced analytics applied to Predictive Maintenance (PdM) help address these challenges by anticipating and preventing equipment failures, thus minimizing downtime, ensuring product quality, and optimizing maintenance costs. (Uzoigwe, 2024)
However, what are the challenges, why is not widely implemented, and what are the barriers?
In some cases, a lack of expertise may hinder the implementation of advanced capabilities, but even with the right skills, a rule-based approach might be preferable as analysts can be more accurate than the model. Sarazin et al. (2019) note that manufacturing plants are dynamic environments, and both insufficient and excessive data heterogeneity can negatively impact machine learning models.
Thomas Gliter et al. (2020) demonstrated that sometimes the collected data cannot support building a reliable model. Their approach involves developing and training a model using a small dataset collected after a machine is newly introduced or after installing new filters. This configuration is often impractical and necessitates continuous model adaptation.
This challenge might be addressed by using diverse datasets and methods. S. Alhuqayl et al. (2024) demonstrated this by employing Linear Regression to predict Remaining Useful Life (RUL) using operational data, Forest Regression to reduce overfitting with multiple decision trees, and Gradient Boosting Regression to sequentially improve accuracy by correcting previous errors. However, having this capability is not a simple thing.
Conclusion
The use of analytics can significantly enhance the accuracy of predicting machinery problems and support maintenance strategies aimed at minimizing downtime, costs, energy consumption, and improving reliability. However, implementing these capabilities is not a simple task. Often, there is a lack of rigor in selecting the appropriate analytics tools, and insufficient understanding can lead to unrealistic expectations about model accuracy. Important metrics, such as Mean Squared Error (MSE), Mean Absolute Error (MAE), and R-squared (R²), are frequently overlooked, which can result in predictive analytics being less effective than rule-based strategies.
A thorough assessment is essential to determine the most suitable application and model. In many cases, a rule-based predictive maintenance (PDM) solution may be the best fit, although the benefits of advanced analytics are clear. By integrating prescriptive capabilities, the potential of analytics in maintenance activities becomes even more substantial.
References
Chen, C. L.-G. (2020). Predictive maintenance using cox proportional hazard deep learning. . Advanced Engineering Informatics, 44, 101054.
IBM. (2024). What is business analytics? Retrieved from https://www.ibm.com/topics/business-analytics?utm_content=SRCWW&p1=Search&p4=43700068106010529&p5=p&p9=58700007556259064&gad_source=1&gclid=CjwKCAjwqMO0BhA8EiwAFTLgIB8W4irnjVIFmWVSA72Nufz9GhYOeiEbSXUd_daRjo9X6oPDGcEDFBoCy9gQAvD_BwE&gclsrc=aw.ds
Lekidis, A., Georgakis, A., Dalamagkas, C., & Papageorgiou, E. (2024). Predictive Maintenance Framework for Fault Detection in Remote Terminal Units. . Forecasting , 239–265.
R Azmi, P., Yusoff, M., & Mohd Sallehud-din, M. (2024). A Review of Predictive Analytics Models in the Oil and Gas Industries. . Sensors.
Saleh Othman Alhuqayl, A. T. (2024). Improving Predictive Maintenance in Industrial Environments via IIoT and Machine Learning. International Journal of Advanced Computer Science and Applications, Vol 15 N4.
Sarazin, A. ,. (2019). Toward information system architecture to support predictive maintenance approach. . In Enterprise interoperability viii , 297-306.
Schmitt, J. B. (2020). Predictive model-based quality inspection using machine learning and edge cloud computing. . Advanced Engineering Informatics, 45, 101101.
Thomas Gittler a, F. S. (2020). Condition monitoring system for machine tool auxiliaries. Elsevier Science Direct .
Uzoigwe, D. O. (2024). Implementing Predictive Maintenance (PdM) Programs in Food and Beverage Manufacturing Facilities. Research Scientific Innovation Society, 103-118.
Comments